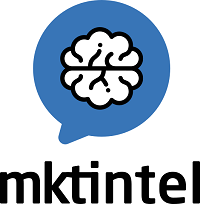
Visit our youtube channel: https://www.youtube.com/user/aacesta/about
Ways to optimise a marketing campaign - André Augusto Cesta.
This article was first published on Linked-in as: Ways to optimise a marketing campaign.; it presents an ontology of ways to optimise marketing campaigns. For first time readers, it may be best to skip now to the ontology table below to get a glimpse of the article and then return here. We assume the practitioner has a set of clear marketing goals at hand, such as: "retain high-value customers"; "lower cost to serve by customer value tier". After defining marketing goals, one would design different competing campaigns that are likely to achieve such goals. The ontology for campaign optimisation is to assist not only campaign design but the whole process from marketing strategy to campaign operations. The ontology should appeal to many practitioners involved in campaign activities: marketing strategists; marketing specialists; business analysts; data scientists; statisticians; marketing operations staff.
I welcome all feedback, questions, contributions, references. Please also share this article; like-it; follow me. If you like this article, there are deep dive videos about it on my channel: https://www.youtube.com/user/aacesta
For optimisation purposes, the most suitable definition of a marketing campaign is that which makes the customer develop a new behaviour which would not have manifested *otherwise. For reference purposes here is the original quote from Linof and Berry: "The goal of a marketing campaign is to change behaviour. In this regard, reaching a prospect who is going to purchase anyway is little more effective than reaching a prospect who will not purchase despite having received the offer"[1].
By "would not have manifested *otherwise" we mean the customer behaviour would not have manifested by the passing of time or by other campaigns targeting him. Campaigns that generate behaviour that customers would have manifested by themselves a few days later; or after being targeted by other treatments; are not achieving much. Several techniques are used to test for campaign effectiveness (1.3). For instance, a design of experiment involving: control groups; champion and challenger; A/B tests or the like[1]. These techniques (1.3) are not covered here. Instead, this article starts from the point where one has an idea about which campaigns to test and how to test them.
On the abstract, this article mentions that one leaps from marketing goals chosen for the company (e.g. retention of high-value customers) to processes and behaviours he/she wants to foister that will lead to those goals (e.g. the value generation process; followed by satisfaction and loyalty behaviours). Subsequently, one leaps to campaigns that will develop those behaviours (e.g. selling a product which is known to raise loyalty and retention rates). Such correlation or causality sequence provided in this paragraph examples is indicated by Fader in his customer centricity work [2].
When the goal is to boost product sales, it is all much straightforward to leap from goals to campaigns, but companies are starting to realise that sales are just part of the full scope of business and marketing performance optimisation game. Customer value management would be a place to start looking at this broader picture[2][3][4]. The path from campaigns to goals passes through the customer's heart before they go through the customer wallet.
Traversing from goals to potential campaigns is not the only way. One can take the reverse path with attribution models. That is: one can analyse a pool of seemingly random campaign variations, and through for instance control groups, champion and challenger, model sensitivity analysis and moreover attribution models (1.3) find out which campaigns are causing or correlated to achieving the desired marketing goals (i.e. to which campaigns we can attribute the results achieved).
The figure below pictures the marketing process as an experimental process. This "1. Test; 2. Learn; 3. Adjust" process proposed by the British IDM (Institute for Direct Marketing) shows the way down from strategy to operations; and the way up from operations back to strategy (we mentioned both paths above). Management wise, such processes are trimmed down versions of Deming’s PDCA (plan, do, check, act). Deming - being a statistician - surely had the design of experiments and statistical process control in mind when designing the PDCA.
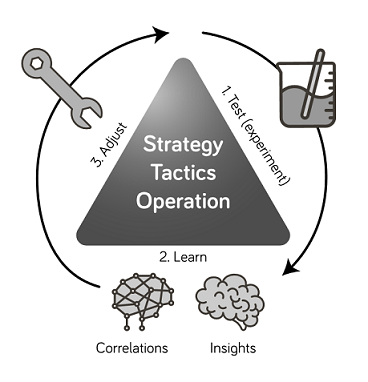
After establishing attribution, one can indeed boost the audience for such campaigns, but even more important: one can look at generating variations of the successful campaigns, and testing these, much like in evolutionary computing. Only these variations will significantly change the ROI metrics and avoid saturating the market audiences with the same message. In a learning organisation, every campaign variation and its analysis results are saved to avoid wasteful repeating of not successful experiments.
The campaign optimisation ontology - the main subject of this article - will be most useful in this process of working with campaign variations to better fulfil a goal. The ontology is mainly a collection of building blocks to create campaigns that will differ from another significantly, and therefore, experiment with a new way of interacting with the customer.
Imagine the work of a medical researcher designing a new pharmaceutical drug with a particular active principle. How many doses of such medication are needed? At which time of the day? In which potency? Are there side-effects? Which is the best logical sequence of administration with regards to other drugs? To which individuals or population groups would this medicine work best? The work of designing new campaigns is not much different in terms of the scientific approach or optimisation building blocks.
The above paragraphs are a very brief introduction to the overall process of campaign optimisation. The following section explores what optimisations there are. A series of Internet videos explore how each optimisation method can be implemented[6].
Ontology Explanation
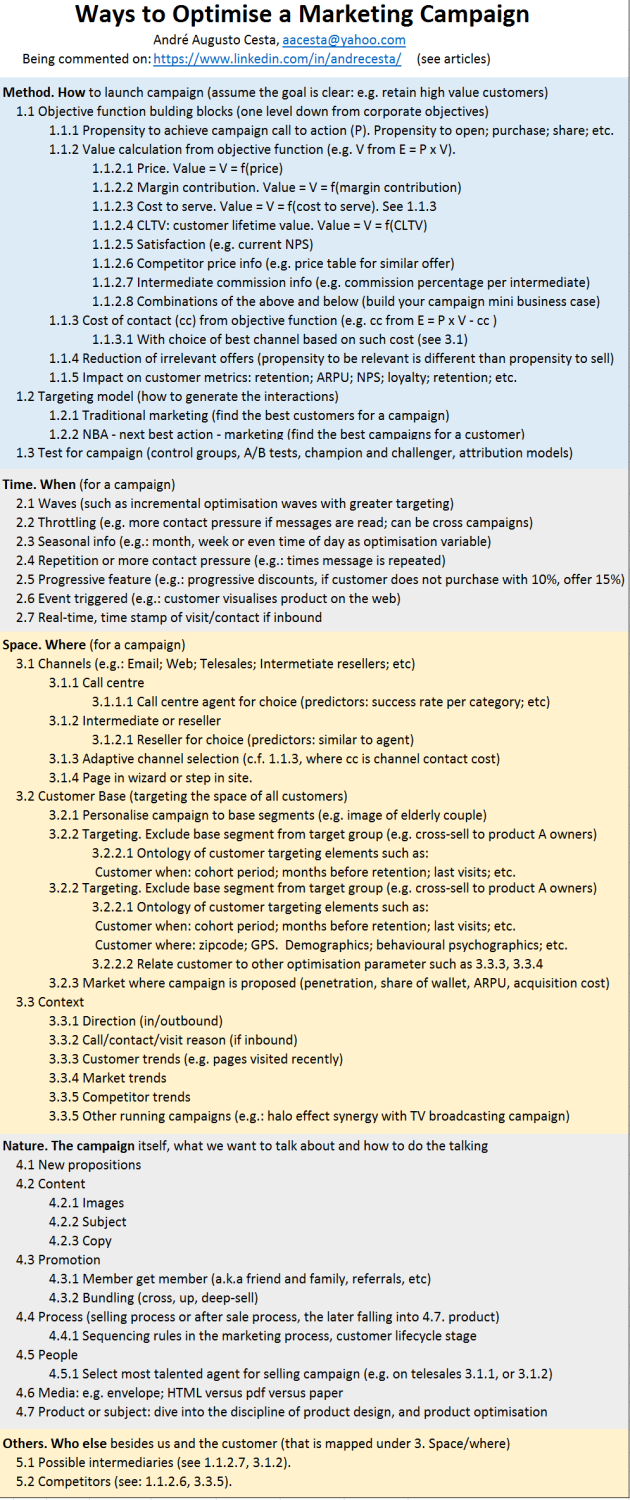
The ontology starts with the objective formula for campaign optimisation (topic 1. Method) and follows with the optimisation variables (topic 2. Time; topic 3. Space). Some optimisation variables are a given, that is: one cannot change them (e.g. time; one cannot change the day of the week today, but one can change the decision to send a campaign today, for a decision to send it on the next Friday).
Regarding “1.1 objective function”. A simple objective function would be (P) or propensity to act on a campaign. In optimisation theory, the objective function expresses the main aim of the model, which is typically maximised. Together with the arbitration rules that can change the objective function applied, they form the rudder of the boat. A wrong function can steer the whole marketing off the path[7].
There are infinite possible objective function formulas; even more so if once considers arbitration rules combining different formula variants (such as: if the customer is complaining do not try to sell). For brevity, this article presents only the main formula building blocks. P or 'propensity for a customer to perform the call to action' is the most typical optimisation formula for traditional marketing (1.1.1), while the most typical call to action in traditional marketing is to propose to the customer to purchase a product (sales).
The ontology presented would like to stimulate practitioners to look at 'calls to action' other than calling the customer to make a purchase. If one considers marketing as “the science and art of exploring, creating, and delivering value…” [Kotler]; then marketing campaigns are to achieve this value not only by selling latent value present in products but also by unlocking value through interaction, nurturing, service, education, convenience and other less conventional types of customer-centric campaigns.
An example of campaign adding value through its interaction would be a bank campaign sent at the right time to sell a short-term loan for a customer who is going to achieve and overdraft situation (e.g. due to automatically detected low balance and scheduled automatic payments – remember internet of things; big data). In the worst case, such a campaign serves as an alert to the customer to top-up his balance with funds from another source. The campaign generates value in any of these two cases.
Another example of a value-added campaign would be to provide the right instructions to use a specific complex product feature a customer has not used yet in his discovery journey. Such nurturing on product usage is made possible with the internet of things monitoring of feature usage, and artificial intelligence driving campaigns sent at the right time to the right customer (NBA or next best action teaching the customer when he is ready to learn). An example for a telecom company would be to send a streaming video on how to use the voicemail after the missed first call. Alternatively, for a bank, this example could be to teach about automatic transfer from a savings account to avoid overdraft once this situation of overdraft is forecasted to happen.
Looking only at propensity to perform a call to action (P) is missing the fact that not all actions or sales are equal. Therefore, V or value is a crucial optimisation variable (1.1.2). Ontology heading 1.1.2, provides several alternatives for value, some of which are still limited, or short-sighted, but still better than optimising campaigns just on P.
Value will often be expressed as a combination of all of the topics under (1.1.2) and even some neighbouring ones. It will be expressed in the long term, using customer margin contribution projections for segments of size one (NBA), this is, in a nutshell, the method behind one of the most exciting forms of calculating V: through customer lifetime value formulas [2], both customer value estimates (for acquisition); or customer value projections (for existing customers).
Price optimisation (1.1.2.1) can be done in several ways. For instance, with adaptive models selecting among many propositions at different price points targeted at different groups and optimised in campaign waves (2.1). A full explanation would require a separate article. This method would dispense with price sensitivity models and would let the adaptive models do the work, on a segment of size one basis. As per previous project experience, these optimisations prove quite effective, especially if combined with competitor price data (1.1.2.6).
P comes together with V in a quite often used formula expressed as E = P x V. It reads as “expected sales value equals propensity to act (as a probability) times expected value from the action (1.2) in for instance Dollars or Euros”. The action quite frequently being a purchase.
Reduction of irrelevant offers (1.1.4) is also interesting for the case where the set of customers for whom this offer is truly relevant is not the same as the set of customers propense to accept. Think of a youngster willing to read educative messages about mortgages, but not yet ready to buy a house. For this purpose, interest must be captured besides the accept or reject of an offer. Ways to capture interest or responses would be the subject of another article.
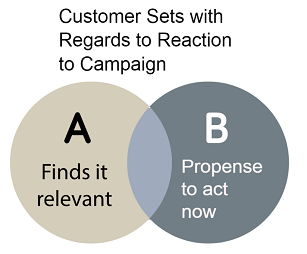
Impact on customer metrics (1.1.5) is a more advanced topic. One can think of it as a suitability check. It may well be the case that a customer is very propense to buy a product, only to dislike it later and churn. Looking at long term customer behaviour can help address these issues. Again, the optimisation function is the rudder of the boat. A good goal or destination would be “to increase the whole customer base value” as opposed to just “generating short-term sales”.
The next section called: “2. Time/when (for a campaign)” starts with a discussion on time-based optimisation, but at its end it returns to the present subject of “objective functions” and optimisation methods where it covers some choices for optimisation method: “Choice 1: optimise campaign variants”; “Choice 2: optimise global parameters”; “Choice 3: let AI do the work”; “Choice 4: deterministic and optimised in a traditional way”.
2. Time/when (for a campaign).
Why would one run a campaign at different times? Because it could be learning to listen to customer responses in (2.1) waves and tune not only propensity models; but also other models such as Bass diffusion for acquisition. Because when the customer is not opening his emails, it could be not only appropriate but polite to throttle communications for a customer busy period (2.2). Because it may be respectful to send less emails if they are not opened. (2.3). Because customer responses may increase for certain seasonal periods (2.4). Because insisting on a message may pay. (2.5) Because changing campaign conversation style with time such as in (whispering, speaking, shouting) may fall in the right ears (2.6). Because events are perhaps the right cue to start a particular campaign, think of a customer looking at a contract cancellation page and being approached with a phone call from the high-value customer retention desk. Finally, because even when there is no clear customer real-time event, just taking into account the time of an inbound visit (2.7) and some context (3.3.3) may allow predicting which campaign is most appropriate to display on a web page.
2.1. Waves. Waves are a key concept behind the 'self-optimising campaign' feature present in a few more advanced marketing automation packages such as Unica (since early releases), and Pega Marketing version 8.2 (self-optimising campaigns are provided out of the box for outbound on version 8.2, and before version 8.2 self-optimising campaigns were always possible programmatically for omnichannel, omnidirectional marketing). Such self-optimising campaigns can target a small number of customers on the first run (say 1000); wait for the customer responses to allow some propensity model tuning, and then proceed to target a larger group of customers in a subsequent run (say 2000).
It is important to distinguish running a self-optimising campaign in waves for traditional outbound (1.2.1) from running it for NBA (1.2.2).
Traditional outbound (1.2.1) is the more straightforward case: one needs to find a mechanism to put all customers in an ordinal sequence, and then target them in waves (the first 1000, the next 2000). The ordinal sequence is usually first established by an initial propensity model or a by a random sequence (to prevent bias). After each wave, the customer ordinal sequence is revised in the light of an improved propensity model and can exclude already targeted customers.
When running NBA (1.2.2) for inbound customers, the need for waves is made redundant by the very pace at which customers come into the channel. Such relatively slow pace ensures that a campaign learns as the customers come along. It is often the case that inbound NBA campaigns are released with a very high starting propensity ensuring they obtain some initial unbiased customer exposure over older campaigns with converged propensity. As customers come by and potentially reject the campaign, its propensity model needs to be revised. Upon revising the model, the initial high starting propensity is blended with the learned propensity, that gains more evidence or weight in the blending after each interaction until the blend converges to the learned propensity. This process causes initially launched campaigns to fall in their NBA rank as time passes until they occupy their place of right among other learned campaigns.
How do traditional outbound campaigns (1.2.1) differ from NBA campaigns for outbound (1.2.2)? Traditional outbound starts with one campaign and chooses the most suitable customers for it, which can be done in waves (see above paragraph on traditional outbound). NBA campaigns for outbound (1.2.2) assumes more than one campaign competing for the customer base. The later is better as one never runs out of customers this way, and all campaigns get a chance to compete for customers to show their value.
NBA for outbound (1.2.2) requires a gradual introduction of new campaigns to the customer base (in waves). Old and learned campaigns are free to target the whole base.
How to introduce new NBA outbound campaigns to the customer base little by little? The metaphor of driving an automobile is quite useful to explain said introduction. One wants to start the new outbound campaign with a high starting propensity, so it gets some exposure. It is started in the first gear to cover a certain small space (or number of customers) and learn from the responses. If one confidently learned, the system revises the propensity models and sets the campaign on second gear for an even larger wave of customer percentiles. If one did not confidently learn, he/she could hit the brakes. By “hitting the brakes” it can be implied: abort experiment and go back to the drawing board.
Also, the wave size can be a function of how much evidence/confidence one obtained on the previous round. That is the wave size can be a function, and it need not be linear. An above linear trend may be what one needs to cover a large customer base quickly in a matter of a few days or waves. The spacing in between the waves may be crucial as there may not be enough time for customers to respond; or the non-responders percentage may be so high that it takes a larger set of targetted customers to build the first version of a campaign's propensity to respond model.
Most packages offering self-optimising campaigns offer them in much more limited rendition than described above. How to programmatically create self-optimising campaigns would however be a subject for a separate article. In a nutshell, programming self-optimising campaigns requires waves. Waves can be formed by a repeatable integer hash function that imprints an order to all customers, and percentiles ranges to delimit waves start and end on this customer base ordered sequence. Then one can proceed to use campaign start date and previous targeted customers as extra parameters to the wave size functions.
Finally waves and being able to cancel campaigns automatically if the adaptive models did not learn enough; are key features behind running champion and challenger experiments; hyper-personalisation experiments in auto-pilot mode.
How about 2.3 Seasonal info? It is easier to explain it with the example of “day of the week” optimisation, which we have implemented for some customers. Some businesses most successful campaigns ran on Fridays or Saturdays when workers have extra free time to read marketing messages. Such higher average success rate on a certain day of the week is no reason to block campaigns from being sent on Mondays as in A/B testing. When doing NBA, it may be that for retirees, Mondays could be a great day as during the weekend they are far too busy caring for the family. How to implement it?[6] Make the day of the week (or month or year for that matter) an optimisation variable and calculate the best combination of day of the week; customer; next best action campaign (the one that achieves higher propensity or any other objective formula). Only send the campaign on its optimal day for the contemplated time horizon (if today does not reach the maximum objective function value, then do not send anything to the customer with regards to this campaign at least).
How about 2.4 repetitions, and to some extent 2.2 throttling?
First, one can decide whether to repeat a campaign with the same communication, or with a slightly different message or copy text. The paragraphs below present four optimisation method choices used to experiment with repetitions:
Choice one: optimise campaign variants. Start by designing two versions of the same campaign, each with a different repetition configuration, and optimising their choice. Choice can be optimised, for instance, via champion and challenger methods (make the choice sticky once it is made). It may be that artificial intelligence ends up using both campaigns well at the same time, by being able to find customers who respond well to each variant (i.e. customers who respond well to repetition; and customers who do not).
Choice two: optimise global parameters. In the above example, the repetition configuration is an attribute of the campaign being run. It could be more interesting to have the repetition configurations - or anything else being experimented with – moving up to become attributes of a whole category of campaigns (e.g. having all retention campaigns tested with 2 or 3 max repetitions allowed per month). Here the count of repetitions is made by adding all campaign interactions in the category. Here, a different campaign on the same category would naturally have a different message. The logic of such global or per category optimisations is a little more complex to implement in the optimisation and arbitration logic. Finally, this choice of optimisation method can also optimise by tailoring repetition (2 or 3 max repetitions) on a per customer basis.
Choice three: let AI do the work. AI, artificial intelligence adaptive propensity models, are a very elegant way to optimise repetitions (and throttling). In order to allow artificial intelligence adaptive models make decisions based on time, it needs to be fed an array of predictors related to repetitions; time; contact pressure; response. Some examples of such predictors: (a). Total number of outbound direction contacts in the last 7, 14, 21 days. (b). Idem for inbound. (c). Number of contacts per direction and category (e.g. retention) in the last 7, 14, 21 days. (d). Was the last email opened? (e). Recency of last communication sent (e.g. 1 day ago). (f). Average historical success rate of campaigns in this category with 1 repetition; 2 repetitions; and so forth.
In the above adaptive model-based optimisation, it is interesting to evaluate the propensity of at least two scenarios here exemplified with a week timeframe. Scenario 1: sending a campaign on the best day this week with the above predictors related to repetitions; Scenario 2: waiting to send a campaign on the best day next week, with slightly different predictors related to repetitions since waiting a week would impact (e). Recency of last communication sent; and many others above. Again, if waiting pays-off in terms of objective function value, one would not send anything this week (nothing at all if we are calculating (e). recency of last communication and other predictors across all campaigns and not per category).
Choice 4: deterministic and optimised traditionally. Determine the number of messages per period (e.g. one educational message per week), run it for a season and then change this number (e.g. one educational message per fortnight). Perform an insight analysis on which method works best and if they work best for all customers or some customer profiles.
It may be interesting to apply the above choices separately to marketing messages. For service messages; and educational messages; it may be interesting to have messages skip queue and be sent any time since the customer may need them immediately (e.g. a message saying “we see you accessed the email filter page but did not configure it, here is a video on how to use it”). One could run a separate optimisation experiment for educational messages, using separate method choices and separate contact pressure allowed.
Finally: while it is easier and much simpler for both marketing and IT to employ one, and only one, of the above choices of optimisation method; there may be situations calling for the combination of choices; or the split of the optimisation space of campaigns (e.g. retention split from acquisition, and education).
3. Space/where (for a campaign)
3.1 New channels added in a "1.2.2 NBA Omnichannel targeting model". Optimise the channel choice? How? See ontology 1.1.3, where the objective function takes into account the cost of contact on a particular channel (cc). In this optimisation approach, one contemplates sending a particular campaign for all channels, and the channel with the highest objective function result wins. Remember: the higher the cost of contact (e.g. a telesales call) the lower the rank of that campaign objective function evaluation. Notice that also the propensity to perform a call to action (P) can also be influenced by the channel as a predictor (e.g. older customers prefer to be called than receiving a short text message). I have done this optimisation quite a few times. The first time for a world bank branch in Australia.
Another type of channel optimisation related to channels aims at potentiating the human skills in selling or servicing within that channel: call centre agents for call contact centre channel; field-sales staff for field sales channel (not present in ontology). We exemplify this technique here with 3.1.1.1 Call centre, but this could equally apply to any channel using people. For some of my customers, I have created an agent/sales-person/Advisor Analytical Record (AAR term I am coining here) which reflects the predictors of success rate on sales per product category; and also the agent's price negotiation efficiency per product category (e.g. able to sell without discount or not). This AAR analytical record is then combined with the customer analytical record (CAR) and the portfolio of offers to optimise what are the best offers for each customer interaction being served by a known agent. If the agent is free to change (because we have not yet connected the phone call), then we are able to - at call connect time, or telemarketing job assignment time - select the agent-offer combination that achieves the highest objective function value. This type of optimisation also falls in the ontology category 4.5 of optimising "people", where it is shortly described with other nuances including "organisations" or sets of "people".
Some firms also select their best agents/advisors for exclusive service to high-value customers, this is both management practice and an optimisation (optimise per customer value tier). It hints on the broader aspects of contact centre digital transformation where agent success rates are equated to talent; this talent is recognized/rewarded, and the company aims at cloning this talent through formal training programs.
Regarding optimising "3. where" to show a banner on a multi-page wizard or process (3.1.4). Beginners should start by placing the optimised banner, or "next best action rank of propositions" on a fixed page such as the last page of the process where the customer has finished his goal and is ready for some other actions. For intermediate and advanced adopters one can simply generate 3 versions of a process with 3 different positions of the offers and see what works best or keep the 3 versions of a process as personalisation.
3.2. Customer base optimisation for a campaign (3.2 targeting). The literature of propensity models has explored this subject extensively [1]. This work focuses, therefore, on the topic of personalisation (3.2.1) where the campaign variant is optimised to the customer, and not the converse (3.2.2). When running, for instance, an A/B variant test in traditional outbound marketing, one will look at eliminating the looser campaign (A or B). With NBA this is not the case and one will typically end-up with both A and B as winners, just for different customer profiles. The chart below shows how minor personalisation gains add up as more campaign variants are introduced (campaign A’, A’’).
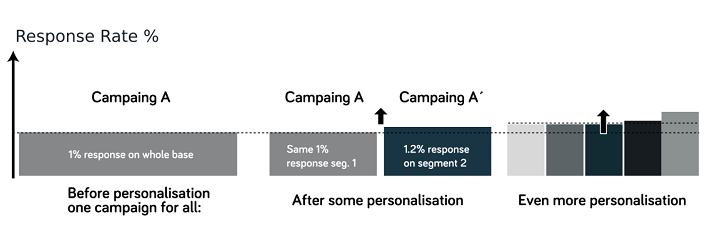
Still within (3.2) targeting, one could address the subjects of profiling and retargeting which are forms optimising customer interactions (profile customers coming from another place, or coming into a company’s customer base; retarget customers going to another channel or place).
Profiling is typically used to gauge customer quality and acquire customers by quality and not quantity. The acquisition of high quality, high-value customers, is one of the main strategic marketing concerns according to the IDM. Some APIs for marketing such as Google ad-words allows profiling (find and target customers like this one example). Profiling can also be done on one’s own website by looking at the referrer variable (indicating which site sent a visitor to one’s own site) and other variables (browser type, and others). A full description of this method would require a full article.
Retargeting can be useful to start a journey on one’s channels (owned media) and continue or rescue it on paid media. For instance: high-value customer abandons his shopping cart; is retargeted with banners for products left in the shopping cart while surfing paid media. A thorough description would require a separate article.
Contextual data (3.3) can be treated along the same lines of customer data (3.2.2.1). That is: it can be fed as predictors to propensity models. Proper data-wrangling may be required as usual.
Optimising the nature of a campaign (4) or what we want to talk about and how to talk it. This is quite often one of the most attempted ways to optimise campaigns. It can be very successful if done in a framework of omnichannel NBA marketing, and a learning organisation focused on a laboratory approach to the data-driven marketing process. Using NBA with adaptive models to optimise the combination of subject, images, header, title of messages on a per-customer basis is far superior to multivariate testing MVT/AB testing which optimizes only one champion message that ends-up being served to customers.
As mentioned before, the connection in between the business objective (e.g. retention) and the campaigns that will achieve that objective is not always straightforward. After enough insight analysis and attribution models, it may turn out that one of the most effective ways to drive retention for a complex product - such as online accounting system - is to monitor which features the user learns, and then send educational videos for the features he is most likely to learn next. Alternatively, it may turn out that in order to drive retention; the product suitability (such as a mortgage of the right size or a calling plan with the right amount of minutes) becomes more important than the propensity to buy a product that is initially attractive, but may later turn out to be unsuitable and unsatisfactory. This indirect connection between campaigns and objectives would require an entire article to explain it. If one assumes not suitable products will increase churn, then the approach exemplified in this video[5] would be an interesting choice.
Ontology topic 4.4 Process: could be considered to cover also multi-step/stage journeys across campaigns and propositions. The transition rules for journeys would take a whole new article to describe as these can be deterministic, probabilistic and one can also look at the probability to complete a journey before starting it, and not just a step in it (sometimes refered to as: Offer Sequencing and with similarities to: Monte Carlo Tree search). If you came here looking for the best page to put a campaign banner on your process or multi-page web wizard go to 3.1.4: Optimize page in wizard or step in process.
Topic 4.5 is a special one. At one telecom customer in London, we looked at empowering physical shop sales agents with tablets that would provide sales recommendations in the form of NBA campaigns. At another telecom in Amsterdam, we optimised the triple: A. campaign; B. most talented telesales agent to sell this campaign; C. customer. Agents were ranked in their success rate for pitching campaigns or product categories. This rank was fed into adaptive models. At an insurance company in Cologne, we generated a different rank of propositions based on the intermediary sales company reselling insurances (3.1.2.1). See also the paragraph above for ontology topic 3.1.1.1 for other ways to optimise the people aspect of campaigns.
When it comes to the targeting model (1.2), one of the most effective ways of running a campaign is to stop doing traditional marketing where one finds the right customers for a campaign, and start doing NBA marketing (1.2.2) where one finds the best campaigns for a customer. NBA unlocks many other ways of optimising a campaign mentioned in this article, many of which are difficult or impossible in traditional marketing (e.g., 1.1.3).
Finally, no matter how honed a firm’s customer-centric marketing is; after optimised to the extreme, it will face the barrier of the company’s products and their shortcomings. Competent marketing departments regularly perform market surveys (penetration, NPS per category[3], benchmarks against competitors[3]), and join such survey results with marketing data to produce actionable insights for product improvement and R&D. One of the most exciting disciplines in this area is "design thinking".
Previous Work
The existing marketing literature covers mostly optimising traditional campaigns (1.2.1) and mainly through what is called the 4Ps or 7Ps of marketing[1]. Depending on the source, these Ps differ, but a non-extensive list is as follows: product, price, promotion, people, place, physical evidence; packaging; processes. Quite a few of these Ps ("product", "packaging") are related to what we classify on the ontology as "4.7. Product optimisation". "Price" optimisation is one P of marketing that would fall into our ontology heading 1.1.2.1 or other neighbour topics.
"People" would fall into ontology headings 3.1.1, 3.1.2. "Promotion" is explicitly categorised under 4.3 and indirectly under 3.2.2 (cross-sell, upsell segmentation), and 3.2.2.1 for customer attribute targeted promotions. "Physical evidence" has more to do with cognitive dissonance where, for instance, the installations of a physical shop are not matching the standard of high quality pitched by marketing messages, this may be applied however to the marketing message in terms of choice of envelope; paper quality; and the like (again under 4.6 Media).
Bibliography
[1] "Data Mining Techniques: For Marketing, Sales, and Customer Relationship Management" - Gordon S. Linof; Michael J. A. Berry
[2] "Customer Centricity: Focus on the Right Customers for Strategic Advantage (Wharton Executive Essentials)" - P. Fader
[3] "Mastering Customer Value Management: The Art and Science of Creating Competitive Advantage" - Ray Kordupleski
[4] "Customer Value Management: Optimizing the Value of the Firm's Customer Base" - Peter Verhoef
[5] Customer value management and CLTV optimisation: https://www.youtube.com/watch?v=WPhLCKlY`RkA&t=37s - André Augusto Cesta
[6] Deep dive into ways to optimise a marketing campaign. Optimising the time a campaign is sent: https://www.youtube.com/watch?v=8FWohGruXgc&list=PLI25Dq0FvDqlIHjFaqpDGVmfv3nCaGkIE - André Augusto Cesta